BlogKnow your sources: AI and the need for communicating authenticity in UX
Recent excitement around AI-generated images and text should prompt UX designers to consider how users will learn to trust content as authentic.
November 9, 2022Unless you have been under a rock, you’ve likely witnessed the Internet’s recent exhilaration with computer-generated images via artificial intelligence (AI) models and their encompassing systems. For instance, OpenAI’s DALL-E creates images that resemble actual photos, digital art, paintings — including styles of Monet, Rembrandt, et al. — all generated within seconds via mere textual descriptions of what the user wishes to see. After submitting the text prompt, the AI takes over and creates multiple versions for you to choose from.
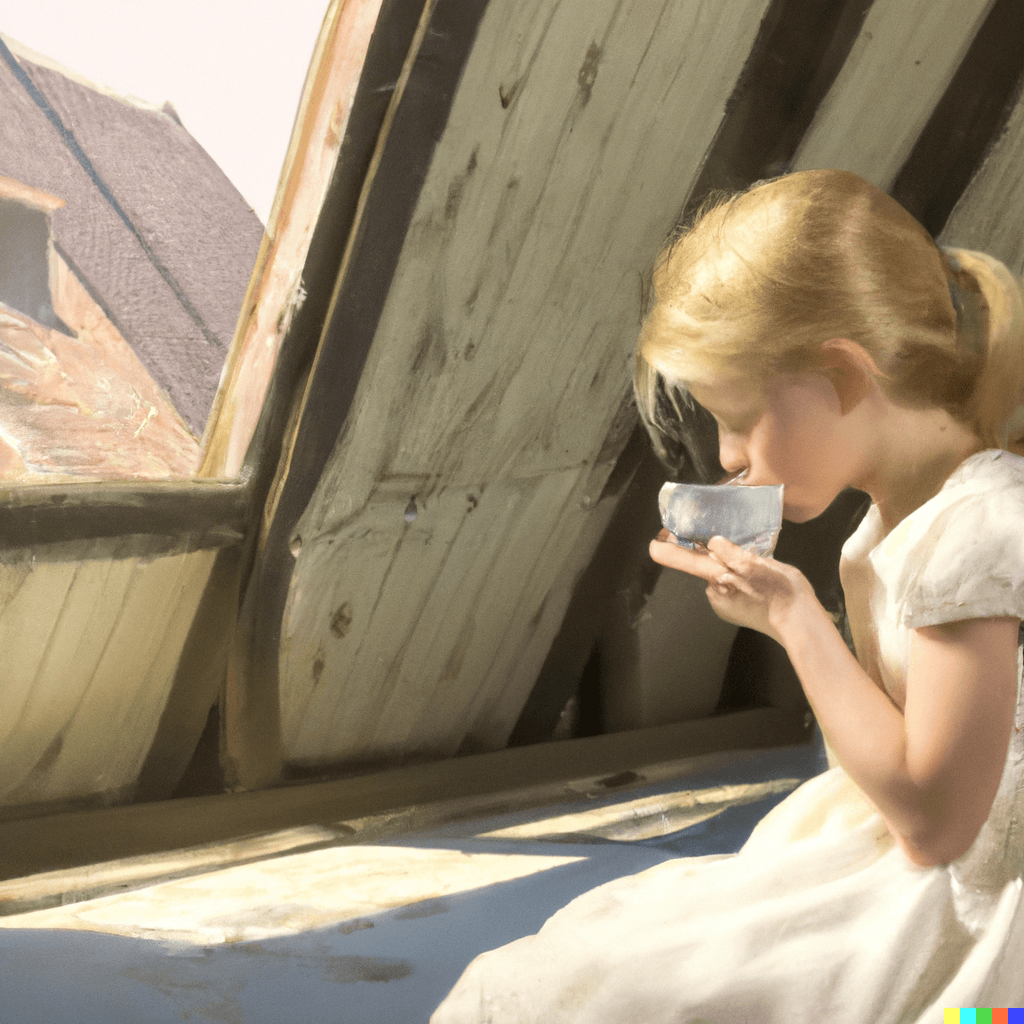
It’s stunning to sit back and realize the generated image was not “found on the Internet”, as a Google image search would return. The image was actually created from scratch by the AI solely based on the text entered. You can feel reasonably confident that the image created by DALL-E or others such tools, like an evening sunset, is the first-ever of its kind to be seen.
Beyond generative-adversarial networks (GANs) — the family of AI models that power DALL-E and others, there are other types too. Generative transformers like OpenAI’s GPT-3 are equally exciting due to their ability to generate text that is, at times, indistinguishable from what a human would write. Beginning with a prompt entered by the user (along with a handful of configuration parameters), the transformer will generate text that is contextual and generally formatted similarly to what has been entered. In fact, the user-entered prompt can give direction to the transformer concerning the style output to produce. See the screenshot below where I indicated the output should be written stylistically as a press release using the bulleted text as a reference:
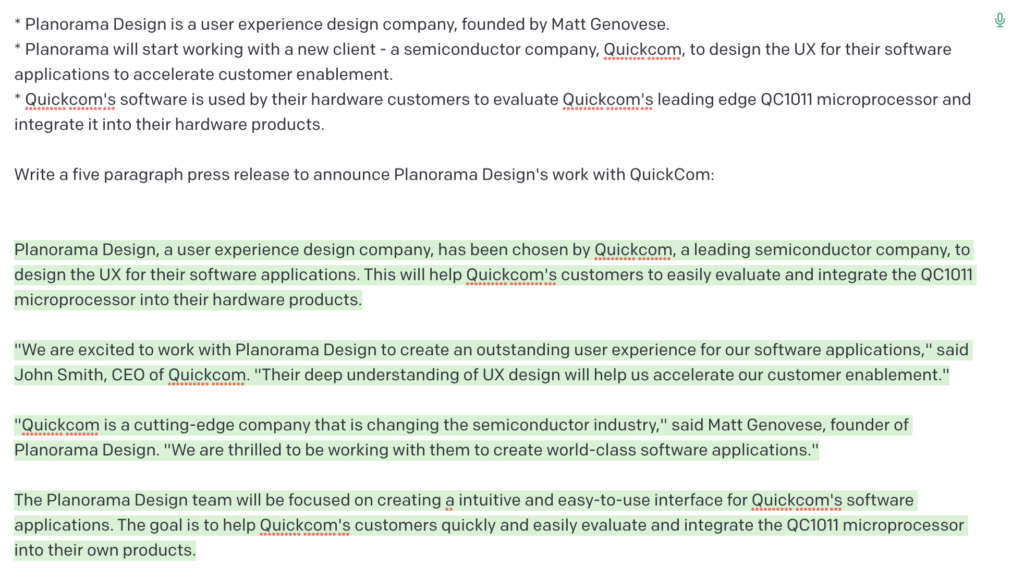
Teachers should be worried…
Deep fake videos have been around for years now, but they required some decent compute performance and know-how to generate. Today though, everything I just discussed is available in the cloud with negligible compute performance requirements on the user’s end, and as easy to use as Google. This powerful AI is available to the masses, and yes, educators should be concerned about just how their students’ essays were written. In fact, we at Planorama wrote a free User Story Generator web application building upon these models as part of our own research in AI-assist with authoring software requirements.
Collectively, we are now faced with a problem: detection. When educators were once concerned about students plagiarizing online content from places like Wikipedia, tools eventually arrived that scanned articles by the thousands to identify text that was more than likely copied and pasted by the student. However, with AI-generated content, this problem is vastly more difficult. Embedded in many AI architectures, there is an integral component that attempts to detect authenticity according to the original prompt. For instance, in GANs, the “A” is for adversarial — meaning, it attempts to discriminate for inauthenticity, or fakeness for what was proposed by the generative AI. And when it does, the GAN continues to generate until the result is no longer detected as such. This is a primary reason why the results of GAN AI’s are so convincing.
While a gross oversimplification, the predicament is this: if an algorithm eventually comes along to detect whether content is AI-generated, the generative AI model would then be improved to incorporate that new algorithmic detection of fakeness. In other words, it’s a cat and mouse game, but the AI will always eventually win because it drives towards producing content that passes all of our tests for authenticity.
Why is this an issue for user experience?
Users of all applications, whether consumer or enterprise, regularly engage with content. Content such as images, text, voice, video, AR/VR/XR — it all derives from somewhere. We take it for granted today, but daily we make contextual assumptions about application’s content, trusting that it’s indeed from the sources we reasonably expect. For instance, photographs or video of a protest are genuine, and new stories are written by actual people. Or a submitted book report essay was actually written by the student. The conundrum I outlined above presents a direct challenge to detecting source authenticity, even inhibiting detection of AI-generated content altogether.
Today, throughout websites or software we use daily, we almost subconsciously scan for trust markers. Consider:
- Affordances stating that another user’s account is authenticated vs. anonymous
- Pad lock icons that represent some type of established trust for login or payment
- Verified purchasers of products who give reviews
- Digitally-signed documents
However, in the future when we see a photo, a video, audio, or article, how will we deem it authentic from a known source, or the source we expect? I anticipate UX design in the future will need to establish common methods to convey a trust that the source of the content is indeed verified as authentic.
Continue reading in part two, Know your sources: AI and the need to validate authenticity
Don't miss out on the latest insights and trends in UX design and AI research! Subscribe to our newsletter.